ΠΡΡΠ³ΠΈΠ΅ ΡΠ°Π±ΠΎΡΡ

ΠΡΠ΅Π΄ΠΏΠΎΠ»ΠΎΠΆΠΈΡΠ΅Π»ΡΠ½ΠΎΡΡΡ ΠΌΠΎΠ³ΡΡ Π²ΡΡΠ°ΠΆΠ°ΡΡ ΠΈ ΡΠ°ΠΊΠΈΠ΅ ΠΌΠΎΠ΄Π°Π»ΡΠ½ΡΠ΅ ΡΠ»ΠΎΠ²Π° ΠΊΠ°ΠΊ perhaps, maybe, probably, possibly. ΠΠ½ΠΎΠ³ΠΈΠ΅ Π»ΠΈΠ½Π³Π²ΠΈΡΡΡ Π³ΠΎΠ²ΠΎΡΡΡ ΠΎ ΠΌΠΎΠ΄Π°Π»ΡΠ½ΡΡ
ΡΠ»ΠΎΠ²Π°Ρ
ΠΊΠ°ΠΊ ΠΎ ΡΠ°ΠΌΠΎΡΡΠΎΡΡΠ΅Π»ΡΠ½ΠΎΠΉ ΡΠ°ΡΡΠΈ ΡΠ΅ΡΠΈ. ΠΡ
ΡΠΈΠ½ΡΠ°ΠΊΡΠΈΡΠ΅ΡΠΊΠ°Ρ ΡΡΠ½ΠΊΡΠΈΡ — ΡΡΠ½ΠΊΡΠΈΡ Π²Π²ΠΎΠ΄Π½ΠΎΠ³ΠΎ ΡΠ»Π΅Π½Π° ΠΏΡΠ΅Π΄Π»ΠΎΠΆΠ΅Π½ΠΈΡ. ΠΠΎΠΏΡΠΎΡ ΠΎ ΠΌΠΎΠ΄Π°Π»ΡΠ½ΡΡ
ΡΠ»ΠΎΠ²Π°Ρ
Π±ΡΠ» Π²ΠΏΠ΅ΡΠ²ΡΠ΅ ΠΏΠΎΡΡΠ°Π²Π»Π΅Π½ ΡΡΡΡΠΊΠΈΠΌΠΈ Π»ΠΈΠ½Π³Π²ΠΈΡΡΠ°ΠΌΠΈ Π² ΠΎΡΠ½ΠΎΡΠ΅Π½ΠΈΠΈ ΡΡΡΡΠΊΠΎΠ³ΠΎ ΡΠ·ΡΠΊΠ°. Π Π·Π°ΡΡΠ±Π΅ΠΆΠ½ΠΎΠΉ Π»ΠΈΠ½Π³Π²ΠΈΡΡΠΈΠΊΠ΅ Π΄Π°Π½Π½ΡΠΉ ΡΠΈΠΏ Π±ΡΠ» ΠΎΡΠΌΠ΅ΡΠ΅Π½, Π½ΠΎ Π½Π΅ Π±ΡΠ»…
ΠΠΈΠΏΠ»ΠΎΠΌΠ½Π°Ρ 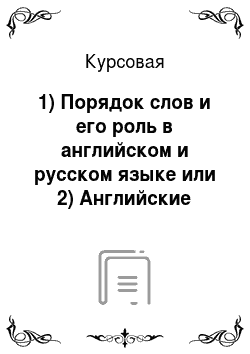
N umerous questions were put to Squadron Leader Worth, who dealt with the fundamental queries with logic and taste, while he brushed aside the frivolous with a quip that met with the hearty approval of most of those present. ΠΠ°ΠΉΠΎΡΡ Π°Π²ΠΈΠ°ΡΠΈΠΈ ΠΠΎΡΡΡ Π±ΡΠ»ΠΎ Π·Π°Π΄Π°Π½ΠΎ ΠΌΠ½ΠΎΠΆΠ΅ΡΡΠ²ΠΎ Π²ΠΎΠΏΡΠΎΡΠΎΠ². ΠΠ½ Π»ΠΎΠ³ΠΈΡΠ½ΠΎ ΠΈ ΡΠ°ΠΊΡΠΈΡΠ½ΠΎ ΠΎΡΠ²Π΅ΡΠ°Π» Π½Π° ΠΏΡΠΈΠ½ΡΠΈΠΏΠΈΠ°Π»ΡΠ½ΡΠ΅ Π²ΠΎΠΏΡΠΎΡΡ ΠΈ ΠΎΡΠ΄Π΅Π»ΡΠ²Π°Π»ΡΡ ΠΎΡ ΠΏΡΡΡΡΡ
Ρ ΠΎΡΡΡΠΎΡΠΌΠΈΠ΅ΠΌ, ΡΠ½ΠΈΡΠΊΠ°Π²ΡΠΈΠΌ ΠΈΡΠΊΡΠ΅Π½Π½Π΅Π΅…
ΠΡΡΡΠΎΠ²Π°Ρ 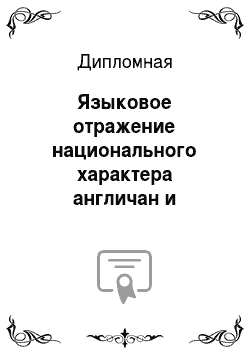
ΠΠΎΠ²ΠΈΠ·Π½Π° ΡΠ°Π±ΠΎΡΡ ΡΠΎΡΡΠΎΠΈΡ Π² ΡΠΎΠΏΠΎΡΡΠ°Π²Π»Π΅Π½ΠΈΠΈ ΡΠ·ΡΠΊΠΎΠ²ΡΡ
ΡΡΠ΅Π΄ΡΡΠ², ΠΈΡΠΏΠΎΠ»ΡΠ·ΡΠ΅ΠΌΡΡ
Π΄Π»Ρ ΡΠ΅Π°Π»ΠΈΠ·Π°ΡΠΈΠΈ Π½Π°ΡΠΈΠΎΠ½Π°Π»ΡΠ½ΠΎΠ³ΠΎ Ρ
Π°ΡΠ°ΠΊΡΠ΅ΡΠ° Π² ΡΠΊΠ°Π·ΠΊΠ°Ρ
ΡΠ°Π·Π½ΡΡ
Π½Π°ΡΠΎΠ΄ΠΎΠ². Π Π°ΡΠΊΡΡΠ²Π°Π΅ΡΡΡ ΠΎΡΠΎΠ±Π΅Π½Π½ΠΎΡΡΠΈ Π½Π°ΡΠΈΠΎΠ½Π°Π»ΡΠ½ΠΎΠ³ΠΎ Ρ
Π°ΡΠ°ΠΊΡΠ΅ΡΠ° ΡΠ°Π·Π½ΡΡ
Π½Π°ΡΠΎΠ΄ΠΎΠ² ΠΈ ΡΡΠ°Π²Π½ΠΈΠ²Π°Π΅ΡΡΡ ΠΎΡΡΠ°ΠΆΠ΅Π½ΠΈΠ΅ Π΄Π°Π½Π½ΠΎΠ³ΠΎ Ρ
Π°ΡΠ°ΠΊΡΠ΅ΡΠ° ΡΠ·ΡΠΊΠΎΠ²ΡΠΌΠΈ ΡΡΠ΅Π΄ΡΡΠ²Π°ΠΌΠΈ. ΠΠ°ΡΡΠΎΡΡΠ°Ρ ΡΠ°Π±ΠΎΡΠ° ΠΏΠΎΡΠ²ΡΡΠ΅Π½Π° ΠΈΠ·ΡΡΠ΅Π½ΠΈΡ ΡΠ·ΡΠΊΠΎΠ²ΠΎΠ³ΠΎ ΠΎΡΡΠ°ΠΆΠ΅Π½ΠΈΡ Π½Π°ΡΠΈΠΎΠ½Π°Π»ΡΠ½ΠΎΠ³ΠΎ Ρ
Π°ΡΠ°ΠΊΡΠ΅ΡΠ° Π°Π½Π³Π»ΠΈΡΠ°Π½ ΠΈ Π²ΠΎΡΡΠΎΡΠ½ΡΡ
ΡΠ»Π°Π²ΡΠ½ Π² Π½Π°ΡΠΎΠ΄Π½ΡΡ
ΡΠΊΠ°Π·ΠΊΠ°Ρ
…
ΠΠΈΠΏΠ»ΠΎΠΌΠ½Π°Ρ 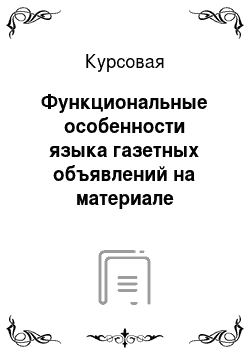
ΠΡΠΈ Π²ΠΊΠ»ΡΡΠ΅Π½ΠΈΠΈ ΡΠ΅Π»Π΅Π²ΠΈΠ·ΠΎΡ ΡΠ°ΠΌ ΠΎΡΡΠ΅Π³ΡΠ»ΠΈΡΡΠ΅Ρ ΠΊΠ°ΡΠ΅ΡΡΠ²ΠΎ ΠΈΠ·ΠΎΠ±ΡΠ°ΠΆΠ΅Π½ΠΈΡ Π² ΡΠΎΠΎΡΠ²Π΅ΡΡΡΠ²ΠΈΠΈ Ρ ΠΎΡΠ²Π΅ΡΠ΅Π½ΠΈΠ΅ΠΌ ΠΈ Π±ΡΠ΄Π΅Ρ ΠΏΡΠΎΠ΄ΠΎΠ»ΠΆΠ°ΡΡ Π΄Π΅Π»Π°ΡΡ ΡΡΠΎ, ΠΏΠΎΠΊΠ° ΡΠ°Π±ΠΎΡΠ°Π΅Ρ. ΠΡΡΠΎΠΊΠΎΠ΅ ΠΊΠ°ΡΠ΅ΡΡΠ²ΠΎ ΠΈΠ·ΠΎΠ±ΡΠ°ΠΆΠ΅Π½ΠΈΡ Π³Π°ΡΠ°Π½ΡΠΈΡΡΠ΅ΡΡΡ, Π΄Π°ΠΆΠ΅ Π΅ΡΠ»ΠΈ Π½Π° ΡΠΊΡΠ°Π½ Π±ΡΠ΄Π΅Ρ ΠΏΠ°Π΄Π°ΡΡ ΡΡΠΊΠΈΠΉ ΡΠΎΠ»Π½Π΅ΡΠ½ΡΠΉ ΡΠ²Π΅Ρ — Π΄Π»Ρ ΡΡΠΎΠ³ΠΎ ΠΏΡΠ΅Π΄ΡΡΠΌΠΎΡΡΠ΅Π½ΠΎ Π°Π½ΡΠΈΠ±Π»ΠΈΠΊΠΎΠ²ΠΎΠ΅ ΠΏΠΎΠΊΡΡΡΠΈΠ΅. ΠΠ° ΠΊΠΎΠΌΡΠΎΡΡ ΠΏΡΠΎΡΠΌΠΎΡΡΠ° Π² Π»ΡΠ±ΡΡ
ΡΡΠ»ΠΎΠ²ΠΈΡΡ
ΡΠ°Π±ΠΎΡΠ°Π΅Ρ Π²Π΅ΡΡ ΡΠΏΠ΅ΠΊΡΡ ΡΠ°Π·ΡΠ°Π±ΠΎΡΠ°Π½Π½ΡΡ
ΡΠΈΡΠΌΠΎΠΉ ΡΠ΅Ρ
Π½ΠΎΠ»ΠΎΠ³ΠΈΠΉ Π² ΠΎΠ±Π»Π°ΡΡΠΈ…
ΠΡΡΡΠΎΠ²Π°Ρ 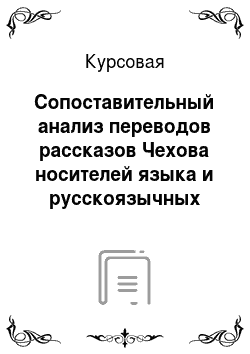
ΠΠΎ Π² ΡΠΎ ΠΆΠ΅ Π²ΡΠ΅ΠΌΡ ΠΏΠΎΠ½ΡΡΠΈΠ΅ «Π±Π΅Π΄Π½ΡΠ³Π°» Π½Π΅ ΠΈΠΌΠ΅Π΅Ρ ΡΠΊΠ²ΠΈΠ²Π°Π»Π΅Π½ΡΠ° Π² Π°Π½Π³Π»ΠΈΠΉΡΠΊΠΎΠΌ ΡΠ·ΡΠΊΠ΅. ΠΡΠΎ Π²Π΅Π΄ΡΡ ΠΊ ΠΏΠΎΡΠ΅ΡΠ΅ ΡΡΠ΄Π° ΡΠΏΠ΅ΡΠΈΡΠΈΡΠ΅ΡΠΊΠΈΡ
ΡΠ΅ΡΡΠ° ΡΠ΅ΠΊΡΡΠ°, ΡΡΠΈΠ»Ρ Π°Π²ΡΠΎΡΠ°. Π Ρ
ΡΠ΄ΠΎΠΆΠ΅ΡΡΠ²Π΅Π½Π½ΠΎΠΌ ΠΏΠ΅ΡΠ΅Π²ΠΎΠ΄Π΅ Π½Π°ΠΈΠ±ΠΎΠ»Π΅Π΅ Π²Π΅Π»ΠΈΠΊΠΎ ΡΠ°Π·Π»ΠΈΡΠΈΠ΅ ΠΌΠ΅ΠΆΠ΄Ρ ΡΠΊΠ²ΠΈΠ²Π°Π»Π΅Π½ΡΠ½ΠΎΡΡΡΡ ΠΈ ΡΠ΅Π½Π½ΠΎΡΡΡΡ ΠΏΠ΅ΡΠ΅Π²ΠΎΠ΄Π°. ΠΠ° ΠΏΠ΅ΡΠ²ΡΠΉ ΠΏΠ»Π°Π½ ΠΏΡΠΈ ΠΏΠ΅ΡΠ΅Π²ΠΎΠ΄Π΅ Π²ΡΡΡΡΠΏΠ°Π΅Ρ Π½Π΅ ΡΠΎΡΠ½ΠΎΠ΅ Π²ΠΎΡΠΏΡΠΎΠΈΠ·Π²Π΅Π΄Π΅Π½ΠΈΠ΅ ΡΠΎΠ΄Π΅ΡΠΆΠ°Π½ΠΈΡ ΠΎΡΠΈΠ³ΠΈΠ½Π°Π»Π°, Π° ΠΎΠ±Π΅ΡΠΏΠ΅ΡΠ΅Π½ΠΈΠ΅ Π²ΡΡΠΎΠΊΠΈΡ
Π»ΠΈΡΠ΅ΡΠ°ΡΡΡΠ½ΡΡ
Π΄ΠΎΡΡΠΎΠΈΠ½ΡΡΠ² Ρ
ΡΠ΄ΠΎΠΆΠ΅ΡΡΠ²Π΅Π½Π½ΠΎΠ³ΠΎ ΡΠ΅ΠΊΡΡΠ°…
ΠΡΡΡΠΎΠ²Π°Ρ 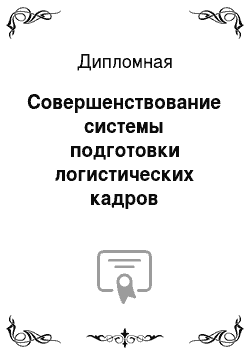
ΠΡΠ½ΠΎΠ²Π½Π°Ρ ΠΏΡΠΈΡΠΈΠ½Π° Π½Π΅Π²ΡΡΠΎΠΊΠΎΠΉ ΠΎΡΠ΄Π°ΡΠΈ ΠΎΡ ΠΎΠ±ΡΡΠ΅Π½ΠΈΡ, ΠΊΠ°ΠΆΡΡΠ΅Π³ΠΎΡΡ Π½Π° ΠΏΠ΅ΡΠ²ΡΠΉ Π²Π·Π³Π»ΡΠ΄ Π±ΠΎΠ»Π΅Π΅ ΡΠ΅ΠΌ ΡΠ΅Π»Π΅ΡΠΎΠΎΠ±ΡΠ°Π·Π½ΡΠΌΠΈ, — Π½Π΅Π΄ΠΎΡΡΠ°ΡΠΎΡΠ½Π°Ρ ΡΡΡΠ΅ΠΊΡΠΈΠ²Π½ΠΎΡΡΡ ΠΈΠ»ΠΈ ΠΎΡΡΡΡΡΡΠ²ΠΈΠ΅ Π²ΠΎΠ²ΡΠ΅ Π΄ΠΈΠ°Π³Π½ΠΎΡΡΠΈΠΊΠΈ ΡΠΎΠ³ΠΎ, ΡΡΠΎ ΠΈΠΌΠ΅Π½Π½ΠΎ Π½Π΅ΠΎΠ±Ρ
ΠΎΠ΄ΠΈΠΌΠΎ ΡΠΎΡΡΡΠ΄Π½ΠΈΠΊΠ°ΠΌ Π΄Π»Ρ ΡΡΠΏΠ΅ΡΠ½ΠΎΠ³ΠΎ Π²ΡΠΏΠΎΠ»Π½Π΅Π½ΠΈΡ ΠΊΠΎΠ½ΠΊΡΠ΅ΡΠ½ΡΡ
ΡΠ°Π±ΠΎΡΠΈΡ
Π·Π°Π΄Π°Ρ Π² ΠΊΠΎΠ½ΠΊΡΠ΅ΡΠ½ΠΎΠΉ ΠΎΡΠ³Π°Π½ΠΈΠ·Π°ΡΠΈΠΈ. ΠΠ»Ρ ΡΠΎΠ³ΠΎ, ΡΡΠΎΠ±Ρ ΠΏΡΠΎΡΠ΅ΡΡ ΠΎΠ±ΡΡΠ΅Π½ΠΈΡ ΠΈ ΡΠ°Π·Π²ΠΈΡΠΈΡ ΠΏΠ΅ΡΡΠΎΠ½Π°Π»Π° Π±ΡΠ» ΠΌΠ°ΠΊΡΠΈΠΌΠ°Π»ΡΠ½ΠΎ ΡΡΡΠ΅ΠΊΡΠΈΠ²Π½ΡΠΌ, ΡΡΠΎΠΈΡ, ΠΏΡΠ΅ΠΆΠ΄Π΅ Π²ΡΠ΅Π³ΠΎ, ΠΎΠΏΡΠ΅Π΄Π΅Π»ΠΈΡΡΡΡ…
ΠΠΈΠΏΠ»ΠΎΠΌΠ½Π°Ρ 
ΠΠΏΡΠΎΡΠ΅ΠΌ, ΠΏΡΠΈ Π±ΠΎΠ»Π΅Π΅ ΡΠΊΡΡΠΏΡΠ»Π΅Π·Π½ΠΎΠΌ Π°Π½Π°Π»ΠΈΠ·Π΅ Π²ΡΡΡΠ½ΠΈΠ»ΠΎΡΡ, ΡΡΠΎ ΡΠ²ΠΎΠΈΠΌ ΡΠ°ΡΠΏΡΠΎΡΡΡΠ°Π½Π΅Π½ΠΈΠ΅ΠΌ ΡΠ°ΠΊΠΈΠ΅ Π½ΠΎΠ²ΠΎΠΎΠ±ΡΠ°Π·ΠΎΠ²Π°Π½ΠΈΡ Π½Π΅ ΠΎΠ±ΡΠ·Π°Π½Ρ ΡΠ»Π΅ΠΊΡΡΠΎΠ½Π½ΠΎΠΉ ΡΡΠ΅Π΄Π΅ ΠΈΠ»ΠΈ ΠΎΡΠΎΠ±Π΅Π½Π½ΠΎΡΡΡΠΌ ΡΠ»Π΅ΠΊΡΡΠΎΠ½Π½ΠΎΠ³ΠΎ Π΄ΠΈΡΠΊΡΡΡΠ°. ΠΡΠΈΠ²Π΅Π΄Π΅Π½Π½ΡΠ΅ ΠΈΠ»Π»ΡΡΡΡΠ°ΡΠΈΠΈ ΠΎΡΠ½ΡΠ΄Ρ Π½Π΅ ΠΈΡΡΠ΅ΡΠΏΡΠ²Π°ΡΡ Π²Π΅ΡΡ ΠΌΠ°ΡΡΠΈΠ² Π½ΠΎΠ²ΠΎΠΉ Π»Π΅ΠΊΡΠΈΠΊΠΈ, Π²ΠΎΡΠ΅Π΄ΡΠ΅ΠΉ Π² Π°ΠΊΡΠΈΠ²Π½ΠΎΠ΅ ΡΠΏΠΎΡΡΠ΅Π±Π»Π΅Π½ΠΈΠ΅, ΠΎΠ΄Π½Π°ΠΊΠΎ ΠΏΡΠΈ ΡΡΠΎΠΌ Π΄Π°ΡΡ Π²Π΅ΡΡΠΌΠ° ΡΠΎΡΠ½ΡΡ ΠΈ Π½Π°Π³Π»ΡΠ΄Π½ΡΡ ΡΠ΅ΠΏΡΠ΅Π·Π΅Π½ΡΠ°ΡΠΈΡ Π»Π΅ΠΊΡΠΈΠΊΠΎΠ»ΠΎΠ³ΠΈΡΠ΅ΡΠΊΠΈΡ
, ΠΈΠ»ΠΈ, Π΅ΡΠ»ΠΈ Π²ΡΡΠ°ΠΆΠ°ΡΡΡΡ ΡΠΎΡΠ½Π΅Π΅, Π½Π΅ΠΎΠ»ΠΎΠ³ΠΈΡΠ΅ΡΠΊΠΈΡ
…
ΠΡΡΡΠΎΠ²Π°Ρ 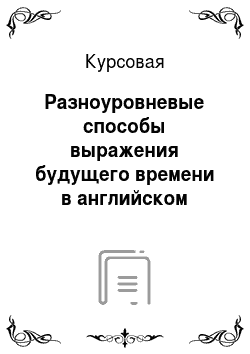
ΠΠ° ΠΎΡΠ½ΠΎΠ²Π°Π½ΠΈΠΈ ΠΏΡΠΎΠ²Π΅Π΄Π΅Π½Π½ΠΎΠ³ΠΎ ΠΈΡΡΠ»Π΅Π΄ΠΎΠ²Π°Π½ΠΈΡ ΠΌΠΎΠΆΠ½ΠΎ ΡΠ΄Π΅Π»Π°ΡΡ ΡΠ»Π΅Π΄ΡΡΡΠΈΠ΅ Π²ΡΠ²ΠΎΠ΄Ρ. ΠΠ»Ρ ΠΏΠΎΠ½ΠΈΠΌΠ°Π½ΠΈΡ ΠΎΡΠΎΠ±Π΅Π½Π½ΠΎΡΡΠ΅ΠΉ ΡΡΠ½ΠΊΡΠΈΠΎΠ½ΠΈΡΠΎΠ²Π°Π½ΠΈΡ Π²ΠΈΠ΄ΠΎΠ²ΡΠ΅ΠΌΠ΅Π½Π½ΠΎΠΉ ΡΠΈΡΡΠ΅ΠΌΡ Π³Π»Π°Π³ΠΎΠ»ΠΎΠ² Π² Π°Π½Π³Π»ΠΈΠΉΡΠΊΠΎΠΌ ΡΠ·ΡΠΊΠ΅ ΠΏΡΠΈΠ½ΡΠΈΠΏΠΈΠ°Π»ΡΠ½ΠΎ Π²Π°ΠΆΠ½ΡΠΌ ΡΠ²Π»ΡΠ΅ΡΡΡ Π²ΡΠ΄Π΅Π»Π΅Π½ΠΈΠ΅ ΠΊΠ°ΡΠ΅Π³ΠΎΡΠΈΠΉ Π²ΡΠ΅ΠΌΠ΅Π½ΠΈ ΠΈ Π²ΠΈΠ΄Π°. ΠΠΈΠ΄ΠΎΠ²ΡΠ΅ΠΌΠ΅Π½Π½ΡΠ΅ ΡΠΎΡΠΌΡ Π³Π»Π°Π³ΠΎΠ»ΠΎΠ² Π² Π°Π½Π³Π»ΠΈΠΉΡΠΊΠΎΠΌ ΡΠ·ΡΠΊΠ΅ ΠΈΠΌΠ΅ΡΡ ΡΡΠ΄ ΠΎΡΠΎΠ±Π΅Π½Π½ΠΎΡΡΠ΅ΠΉ ΠΏΡΠΈ ΠΏΡΠΈΠΌΠ΅Π½Π΅Π½ΠΈΠΈ ΠΈΡ
Π² Ρ
ΡΠ΄ΠΎΠΆΠ΅ΡΡΠ²Π΅Π½Π½ΠΎΠΌ ΠΏΡΠΎΠΈΠ·Π²Π΅Π΄Π΅Π½ΠΈΠΈ. Π ΡΠ°ΡΡΠ½ΠΎΡΡΠΈ, ΡΠ»Π΅Π΄ΡΠ΅Ρ ΡΡΠΈΡΡΠ²Π°ΡΡ ΡΡΠ»ΠΎΠ²Π½ΠΎΡΡΡ…
ΠΡΡΡΠΎΠ²Π°Ρ 
ΠΠΎΠ·Π΄Π΅ΠΉΡΡΠ²ΠΈΠ΅ ΠΎΠ±ΡΠ΅ΡΡΠ²Π΅Π½Π½ΠΎΠΉ ΡΡΡΡΠΊΡΡΡΡ Π½Π° Π²Π°ΡΠΈΠ°ΡΠΈΠ²Π½ΠΎΡΡΡ Π»ΠΈΠ½Π³Π²ΠΈΡΡΠΈΠΊΠΈ Π΄ΠΎΠ»ΠΆΠ½ΠΎ Π°Π½Π°Π»ΠΈΠ·ΠΈΡΠΎΠ²Π°ΡΡΡΡ ΠΏΡΠΈ ΡΡΠΈΡΡΠ²Π°Π½ΠΈΠΈ Π²ΡΠ΅Ρ
ΠΏΡΠΎΠΈΠ·Π²ΠΎΠ΄Π½ΡΡ
ΠΎΡ ΠΊΠ»Π°ΡΡΠΎΠ²ΠΎΠΉ ΡΡΡΡΠΊΡΡΡ ΡΠ»Π΅ΠΌΠ΅Π½ΡΠΎΠ² — ΡΠΎΡΠΈΠ°Π»ΡΠ½ΡΡ
ΡΠ»ΠΎΠ΅Π², ΠΏΡΠΎΡΠ΅ΡΡΠΈΠΎΠ½Π°Π»ΡΠ½ΡΡ
, ΠΊΡΠ»ΡΡΡΡΠ½ΡΡ
ΠΈ ΠΈΠ½ΡΡ
Π³ΡΡΠΏΠΏ, Π²ΠΏΠ»ΠΎΡΡ Π΄ΠΎ ΠΏΠ΅ΡΠ²ΠΈΡΠ½ΡΡ
ΠΊΠΎΠ»Π»Π΅ΠΊΡΠΈΠ²ΠΎΠ² (ΠΌΠ°Π»ΡΡ
Π³ΡΡΠΏΠΏ). ΠΠ΅ΠΏΡΠ΅ΠΌΠ΅Π½Π½ΠΎ Π½ΡΠΆΠ½ΠΎ ΡΡΠΈΡΡΠ²Π°ΡΡ ΠΈ Π²Π»ΠΈΡΠ½ΠΈΠ΅ Π½Π° ΡΠ·ΡΠΊ ΠΊΠΎΠΌΠΏΠΎΠ½Π΅Π½ΡΠΎΠ² ΡΠΎΡΠΈΠΎΠΊΡΠ»ΡΡΡΡΠ½ΡΡ
ΠΈ ΡΠΎΡΠΈΠΎΠΏΡΠΈΡ
ΠΎΠ»ΠΎΠ³ΠΈΡΠ΅ΡΠΊΠΈΡ
ΡΠΈΡΡΠ΅ΠΌ — ΠΎΠ±ΡΠ΅ΡΡΠ²Π΅Π½Π½ΡΡ
ΠΏΡΠ°Π²ΠΈΠ», ΡΡΡΠΎΠ΅Π²…
ΠΡΡΡΠΎΠ²Π°Ρ 
ΠΠ΅ΡΠ΅Π²Π΅Π΄ΠΈΡΠ΅ ΡΠ»Π΅Π΄ΡΡΡΠΈΠ΅ ΠΏΡΠ΅Π΄Π»ΠΎΠΆΠ΅Π½ΠΈΡ Π½Π° ΡΡΡΡΠΊΠΈΠΉ ΡΠ·ΡΠΊ, ΠΎΠ±ΡΠ°ΡΠ°Ρ Π²Π½ΠΈΠΌΠ°Π½ΠΈΠ΅ Π½Π° ΡΠ»ΠΎΠ²Π° ΠΈ ΡΠ»ΠΎΠ²ΠΎΡΠΎΡΠ΅ΡΠ°Π½ΠΈΡ ΠΈΠ· ΡΠ»ΠΎΠ²Π°ΡΠ½ΠΎΠ³ΠΎ ΠΌΠΈΠ½ΠΈΠΌΡΠΌΠ° ΠΊ ΡΠ΅ΠΊΡΡΡ Demand and Supply. ΠΠ΅ΡΠ΅Π²Π΅Π΄ΠΈΡΠ΅ ΠΏΡΠ΅Π΄Π»ΠΎΠΆΠ΅Π½ΠΈΡ Π½Π° ΡΡΡΡΠΊΠΈΠΉ ΡΠ·ΡΠΊ, ΠΎΠ±ΡΠ°ΡΠ°Ρ Π²Π½ΠΈΠΌΠ°Π½ΠΈΠ΅ Π½Π° ΡΠ°Π·Π»ΠΈΡΠ½ΡΠ΅ Π·Π½Π°ΡΠ΅Π½ΠΈΡ ΡΠ»ΠΎΠ²Π° as ΠΈ ΡΠΎΡΠ΅ΡΠ°Π½ΠΈΠΉ Ρ Π½ΠΈΠΌ. Π‘ΠΎΡΡΠ°Π²ΡΡΠ΅ Π²ΡΠ΅ Π²ΠΎΠ·ΠΌΠΎΠΆΠ½ΡΠ΅ ΡΠ»ΠΎΠ²ΠΎΡΠΎΡΠ΅ΡΠ°Π½ΠΈΡ ΠΈΠ· ΡΠ»ΠΎΠ² Π² ΠΏΡΠ°Π²ΠΎΠΉ ΠΈ Π»Π΅Π²ΠΎΠΉ ΠΊΠΎΠ»ΠΎΠ½ΠΊΠ΅ ΠΈ ΠΏΠ΅ΡΠ΅Π²Π΅Π΄ΠΈΡΠ΅ ΠΈΡ
Π½Π° ΡΡΡΡΠΊΠΈΠΉ ΡΠ·ΡΠΊ. ΠΠ΅ΡΠ΅Π²Π΅Π΄ΠΈΡΠ΅ ΡΠ»Π΅Π΄ΡΡΡΠΈΠ΅ ΠΏΡΠ΅Π΄Π»ΠΎΠΆΠ΅Π½ΠΈΡ Π½Π° ΡΡΡΡΠΊΠΈΠΉ…
ΠΠΎΠ½ΡΡΠΎΠ»ΡΠ½Π°Ρ 
ΠΠ½Π°Π»ΠΈΠ· ΡΠ°ΠΊΡΠΈΡΠ΅ΡΠΊΠΎΠ³ΠΎ ΠΌΠ°ΡΠ΅ΡΠΈΠ°Π»Π° ΠΏΡΠΎΠ΄Π΅ΠΌΠΎΠ½ΡΡΡΠΈΡΠΎΠ²Π°Π», ΡΡΠΎ ΠΈΡΠΏΠΎΠ»ΡΠ·ΠΎΠ²Π°Π½ΠΈΠ΅ ΡΠ°ΠΊΠΈΡ
ΡΡΠ΅Π΄ΡΡΠ² ΠΈ ΠΌΠ΅ΡΠΎΠ΄ΠΈΠΊ ΠΎΡΠ΄Π΅Π»Π΅Π½ΠΈΡ ΠΊΠΎΠΌΠΌΡΠ½ΠΈΠΊΠ°ΡΠΈΠ²Π½ΠΎΠ³ΠΎ ΡΠ΅Π½ΡΡΠ° Π²ΡΡΠ°ΠΆΠ΅Π½ΠΈΡ, ΠΊΠ°ΠΊ ΡΠ΅ΠΌΠ°ΡΠΈΠ·Π°ΡΠΎΡΡ, Π² ΡΠΎΠΏΠΎΡΡΠ°Π²Π»ΡΠ΅ΠΌΡΡ
ΡΠ»ΠΎΠ³Π°Ρ
Π·Π½Π°ΡΠΈΡΠ΅Π»ΡΠ½ΠΎ Π½Π΅ ΠΎΡΠ»ΠΈΡΠ°Π΅ΡΡΡ. Π Π²ΠΊΠ½ΠΈΠ³Π°, ΠΈ Π² ΠΈΠ½ΠΎΠΌ ΡΠ·ΡΠΊΠ΅ Π² ΡΠ²ΠΎΠΉΡΡΠ²Π΅ ΡΠ΅ΠΌΠ°ΡΠΈΠ·Π°ΡΠΎΡΠΎΠ² ΡΠΏΠΎΡΡΠ΅Π±Π»ΡΡΡΡΡ — ΠΏΠΎΠ²ΡΡΠΈΡΠ΅Π»ΡΠ½ΡΠ΅ Π½Π°ΡΠ΅ΡΠΈΡ ΠΈ ΡΠ°ΡΡΠΈΡΠΊΠΈ. Π Π² Π°Π½Π³Π»ΠΈΠΉΡΠΊΠΎΠΌ, ΠΈ Π² ΡΡΡΡΠΊΠΎΠΌ ΡΠ·ΡΠΊΠ΅ Π΄Π»Ρ ΠΎΡΠ΄Π΅Π»Π΅Π½ΠΈΡ ΠΊΠΎΠΌΠΌΡΠ½ΠΈΠΊΠ°ΡΠΈΠ²Π½ΠΎ Π²Π°ΠΆΠ½ΠΎΠΉ ΠΈΠ½ΡΠΎΡΠΌΠ°ΡΠΈΠΈ Π΅ΡΠ΅…
ΠΡΡΡΠΎΠ²Π°Ρ 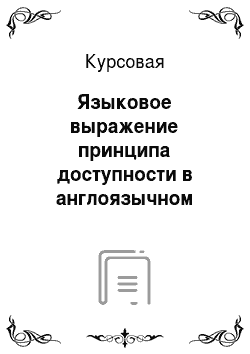
ΠΡΠΎΡΠΈΠ²ΠΎΠΏΠΎΡΡΠ°Π²Π»Π΅Π½ΠΈΠ΅ ΠΆΡΡΠ½Π°Π»ΠΈΡΡΠΎΠΌ ΠΌΠ΅ΡΠΎΠ½ΠΈΠΌΠΈΡΠ΅ΡΠΊΠΈ ΠΏΠ΅ΡΠ΅ΠΎΡΠΌΡΡΠ»Π΅Π½Π½ΡΡ
ΠΏΡΠ΅Π΄ΠΌΠ΅ΡΠΎΠ² Π±ΡΡΠ° ΠΎΠ±ΡΡΠ»ΠΎΠ²Π»Π΅Π½ΠΎ Π½Π΅ ΡΡΠΈΠΌΡΠ»ΡΡΠΈΠ΅ΠΉ ΠΊ ΠΏΠΎΠΊΡΠΏΠΊΠ΅ ΡΠ΅Π»Π΅Π²ΠΈΠ·ΠΎΡΠ° (The TV) ΠΈΠ»ΠΈ Ρ
ΠΎΠ»ΠΎΠ΄ΠΈΠ»ΡΠ½ΠΈΠΊΠ° (the Fridge), Π° ΠΆΠ΅Π»Π°Π½ΠΈΠ΅ΠΌ ΠΏΠΎΠ½ΡΡΡ Π΄Π°Π»ΡΠ½Π΅ΠΉΡΠ΅Π΅ ΡΠ°Π·Π²ΠΈΡΠΈΠ΅ ΠΏΠΎΠ»ΠΈΡΠΈΡΠ΅ΡΠΊΠΎΠΉ ΡΠΈΡΡΠ°ΡΠΈΠΈ Π² Π ΠΎΡΡΠΈΠΈ: ΠΎΡΡΠ°Π½ΡΡΡΡ Π»ΠΈ Π΄Π°Π»ΡΡΠ΅ ΡΡΠ΄ΠΎΠ²ΡΠ΅ ΡΠΎΡΡΠΈΡΠ½Π΅ ΠΏΡΠ΅Π΄Π°Π½Π½ΡΠΌΠΈ ΡΠ²ΠΎΠ΅ΠΌΡ ΠΏΡΠ΅Π·ΠΈΠ΄Π΅Π½ΡΡ, Π±ΡΠ΄ΡΡ ΠΈ Π΄Π°Π»ΡΡΠ΅ ΠΏΠΎΠΊΠΎΡΠ½ΠΎ ΡΠΌΠΎΡΡΠ΅ΡΡ ΡΠ΅Π»Π΅Π²ΠΈΠ·ΠΎΡ ΠΈ ΠΎΠ΄ΠΎΠ±ΡΡΡΡ ΡΠ°ΠΊΡΠΈΠΊΡ ΠΡΠ΅ΠΌΠ»Ρ ΠΈΠ»ΠΈ ΠΆΠ΅ ΠΊΡΠΈΡΠΈΡΠ΅ΡΠΊΠΈ ΠΎΡΠ΅Π½ΡΡ…
ΠΡΡΡΠΎΠ²Π°Ρ 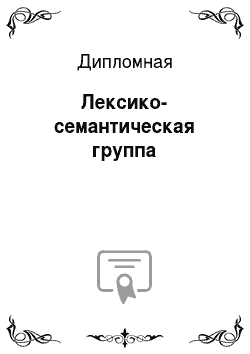
Π―Π²Π»ΡΡΡΡ ΡΠΏΠΎΡΠΎΠ±ΠΎΠΌ ΡΠΎΡΠΌΠΈΡΠΎΠ²Π°Π½ΠΈΡ Π½ΠΎΠ²ΡΡ
ΠΊΠΎΠ½ΡΠ΅ΠΏΡΠΎΠ² ΠΏΡΠΈ ΠΏΠΎΠΌΠΎΡΠΈ ΡΠ»ΠΎΠ², ΡΠΆΠ΅ ΠΈΠΌΠ΅ΡΡΠΈΡ
ΡΡ Π² Π΄Π°Π½Π½ΠΎΠΉ ΡΠ·ΡΠΊΠΎΠ²ΠΎΠΉ ΠΊΠ°ΡΡΠΈΠ½Π΅ ΠΌΠΈΡΠ°, ΠΌΠ΅ΡΠ°ΡΠΎΡΠ° ΠΎΡΠΈΠ΅Π½ΡΠΈΡΠΎΠ²Π°Π½Π° Π½Π° Π΄Π²ΠΎΠΉΡΡΠ²Π΅Π½Π½ΠΎΡΡΡ ΡΠ²ΠΎΠ΅ΠΉ ΡΠΎΡΠΌΡ, ΡΠΎΠ΄Π΅ΡΠΆΠ°Π½ΠΈΡ ΠΈ ΠΏΠΎΠ½ΠΈΠΌΠ°Π½ΠΈΡ. ΠΡΠΏΠΎΠ»ΡΠ·ΡΡ ΡΡ ΠΈΠ»ΠΈ ΠΈΠ½ΡΡ ΠΌΠ΅ΡΠ°ΡΠΎΡΡ Π²ΠΏΠ΅ΡΠ²ΡΠ΅, Π°Π²ΡΠΎΡ ΡΠΎΠ³ΠΎ ΠΈΠ»ΠΈ ΠΈΠ½ΠΎΠ³ΠΎ ΡΠ΅ΠΊΡΡΠ° ΠΎΠ±Π΅ΡΠΏΠ΅ΡΠΈΠ²Π°Π΅Ρ Π²ΠΎΠ·ΠΌΠΎΠΆΠ½ΠΎΡΡΡ Π²ΡΠ±ΠΎΡΠ° ΠΈΠΌΠ΅Π½ΠΈ, ΠΎΡΠ΄Π°Π»Π΅Π½Π½ΠΎΠ³ΠΎ ΠΏΠΎ ΡΠΎΠ΄Π΅ΡΠΆΠ°Π½ΠΈΡ ΠΈ ΡΠΎΠ΄ΠΎ-Π²ΠΈΠ΄ΠΎΠ²ΡΠΌ ΡΠ°Π·Π»ΠΈΡΠΈΡΠΌ; ΠΎΠ±Π΅ΡΠΏΠ΅ΡΠΈΠ²Π°Π΅Ρ ΡΡΠ±ΡΠ΅ΠΊΡΠΈΠ²Π½ΠΎΡΡΡ Π²ΠΊΠ»ΡΡΠ°Π΅ΠΌΡΡ
Π² ΠΈΠ½ΡΠ΅ΡΠ°ΠΊΡΠΈΡ…
ΠΠΈΠΏΠ»ΠΎΠΌΠ½Π°Ρ 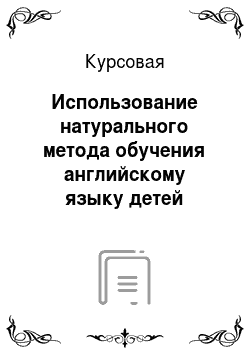
ΠΠ° ΠΎΡΠ½ΠΎΠ²Π°Π½ΠΈΠΈ ΠΏΡΠΎΠ²Π΅Π΄Π΅Π½Π½ΠΎΠ³ΠΎ ΠΈΡΡΠ»Π΅Π΄ΠΎΠ²Π°Π½ΠΈΡ ΠΌΠΎΠΆΠ½ΠΎ ΡΠ΄Π΅Π»Π°ΡΡ ΡΠ»Π΅Π΄ΡΡΡΠΈΠ΅ Π²ΡΠ²ΠΎΠ΄Ρ. ΠΠ°ΡΡΡΠ°Π»ΡΠ½ΡΠΉ ΠΌΠ΅ΡΠΎΠ΄ ΡΠ²Π»ΡΠ΅ΡΡΡ ΠΎΠ΄Π½ΠΈΠΌ ΠΈΠ· Π½Π°ΠΈΠ±ΠΎΠ»Π΅Π΅ ΡΠ°Π½Π½ΠΈΡ
ΠΌΠ΅ΡΠΎΠ΄ΠΎΠ² ΠΎΠ±ΡΡΠ΅Π½ΠΈΡ ΠΈΠ½ΠΎΡΡΡΠ°Π½Π½ΡΠΌ ΡΠ·ΡΠΊΠ°ΠΌ. Π Π΄Π°Π½Π½ΠΎΠΌ ΠΌΠ΅ΡΠΎΠ΄Π΅ ΠΌΠΎΠΆΠ½ΠΎ Π²ΡΠ΄Π΅Π»ΠΈΡΡ, ΠΊΠΎΠ½Π΅ΡΠ½ΠΎ ΠΆΠ΅, ΠΊΠ°ΠΊ ΠΏΠΎΠ»ΠΎΠΆΠΈΡΠ΅Π»ΡΠ½ΡΠ΅, ΡΠ°ΠΊ ΠΈ ΠΎΡΡΠΈΡΠ°ΡΠ΅Π»ΡΠ½ΡΠ΅ ΠΌΠΎΠΌΠ΅Π½ΡΡ, Π½ΠΎ, ΡΠ΅ΠΌ Π½Π΅ ΠΌΠ΅Π½Π΅Π΅, Π½Π°ΡΡΡΠ°Π»ΡΠ½ΡΠΉ ΠΌΠ΅ΡΠΎΠ΄ ΠΏΠΎΠΌΠΎΠ³Π°Π΅Ρ ΡΠ΅ΡΠ°ΡΡ ΠΎΠ΄Π½Ρ ΠΈΠ· Π³Π»Π°Π²Π½ΡΡ
Π·Π°Π΄Π°Ρ Π² ΠΎΠ±ΡΡΠ΅Π½ΠΈΠΈ ΠΈΠ½ΠΎΡΡΡΠ°Π½Π½ΠΎΠΌΡ ΡΠ·ΡΠΊΡ — ΡΠ΅ΡΡ ΠΈΠ΄Π΅Ρ ΠΎ ΡΠ΅ΡΠ΅Π²ΠΎΠΉ ΡΠ°ΡΠΊΡΠ΅ΠΏΠΎΡΠ΅Π½Π½ΠΎΡΡΠΈ…
ΠΡΡΡΠΎΠ²Π°Ρ 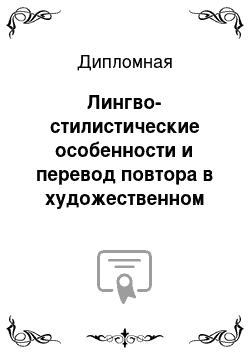
ΠΡΠΎΠ±Π»Π΅ΠΌΠ° Π°Π΄Π΅ΠΊΠ²Π°ΡΠ½ΠΎΠΉ ΠΈΠ½ΡΠ΅ΡΠΏΡΠ΅ΡΠ°ΡΠΈΠΈ ΠΏΠ΅ΡΠ΅Π²ΠΎΠ΄ΡΠΈΠΊΠΎΠΌ ΡΠ΅ΠΊΡΡΠ° ΠΈΡΡΠ»Π΅Π΄ΡΠ΅ΠΌΡΡ
Π½Π°ΠΌΠΈ ΡΠ΅ΠΊΡΡΠΎΠ² ΠΎΠΊΠ°Π·ΡΠ²Π°Π΅ΡΡΡ ΠΎΠ±ΡΡΠ»ΠΎΠ²Π»Π΅Π½Π½ΠΎΠΉ Π½Π΅ Π±ΡΠΊΠ²Π°Π»ΡΠ½ΡΠΌ ΠΏΠ΅ΡΠ΅Π²ΠΎΠ΄ΠΎΠΌ ΡΠ΅ΠΊΡΡΠ° ΠΏΡΡΠ΅ΠΌ ΠΎΠ±Π½Π°ΡΡΠΆΠ΅Π½ΠΈΡ ΡΠΎΠΎΡΠ²Π΅ΡΡΡΠ²ΠΈΡ Π΄Π»Ρ ΠΊΠ°ΠΆΠ΄ΠΎΠΉ Π΅Π΄ΠΈΠ½ΠΈΡΡ ΠΏΠ΅ΡΠ΅Π²ΠΎΠ΄Π°, Π° ΠΏΡΡΠ΅ΠΌ ΠΏΠ΅ΡΠ΅ΠΈΡΡΠΎΠ»ΠΊΠΎΠ²Π°Π½ΠΈΡ ΡΠΌΡΡΠ»Π° ΠΏΡΠΈ ΠΏΠΎΠΌΠΎΡΠΈ Π΄ΡΡΠ³ΠΎΠ³ΠΎ Π»Π΅ΠΊΡΠΈΡΠ΅ΡΠΊΠΎΠ³ΠΎ ΠΌΠ°ΡΠ΅ΡΠΈΠ°Π»Π° Ρ Π΄ΡΡΠ³ΠΎΠΉ Π»ΠΎΠ³ΠΈΡΠ΅ΡΠΊΠΎΠΉ ΠΏΠΎΡΠ»Π΅Π΄ΠΎΠ²Π°ΡΠ΅Π»ΡΠ½ΠΎΡΡΡΡ, ΠΊΠΎΡΠΎΡΠ°Ρ ΠΎΠ±ΡΡΠ»Π°Π²Π»ΠΈΠ²Π°Π΅Ρ ΡΠ°ΡΡΠΎΠ΅ ΠΏΡΠΈΠΌΠ΅Π½Π΅Π½ΠΈΠ΅ Π³ΡΠ°ΠΌΠΌΠ°ΡΠΈΡΠ΅ΡΠΊΠΈΡ
ΠΏΡΠΈΠ΅ΠΌΠΎΠ² ΠΏΠ΅ΡΠ΅Π²ΠΎΠ΄Π°, Π²Π½ΠΈΠΌΠ°Π½ΠΈΠ΅ΠΌ…
ΠΠΈΠΏΠ»ΠΎΠΌΠ½Π°Ρ